Research Lifecycle. Based on: Nicholas, David & Rowlands, Ian & Wamae, Deanna. (2012). Charleston Conference Observatory: Are Social Media Impacting in Research?. https://doi.org/10.5703/1288284314807. p. 16
The “research data lifecycle” (RDL), pictured below, is closely related. On the one hand, the RDL tracks the research lifecycle, highlighting your continuous interaction with your data over the arc of a research project. At every point in a research project — from your initial ideas about what data you’ll need to support your claims, through collecting and analyzing those data, through sharing them when you publish based on them, through preserving them for yourself and others — you are working with your data.
On the other hand, the RDL extends beyond the research lifecycle (i.e., beyond the dissemination of research results). It also involves ensuring the independent existence, accessibility, discoverability and longevity of all of the data generated in association with a research project after its conclusion.
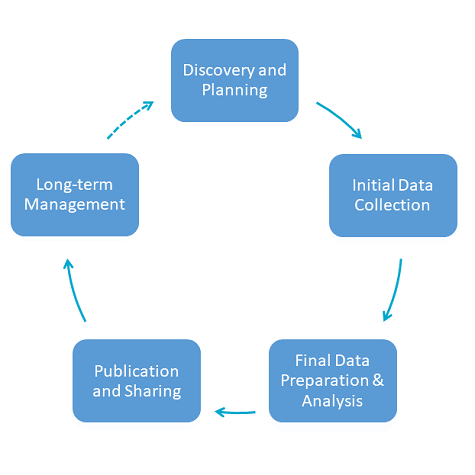
Research Data Lifecycle. Based on: Green, Ann G., and Myron P. Gutmann. (2007) “Building Partnerships among Social Science Researchers, Institution-based Repositories, and Domain Specific Data Archives.” OCLC Systems and Services: International Digital Library Perspectives. 23: 35-53. http://doi.org/10.1108/10650750710720757
As you think through and execute your project, keep the RDL in mind (or even physically in front of you: here is a pdf version to print out, and make sure that you are taking good care of your data at every step of the way!
Exercise
You and the Research Data Lifeycle
- Take another close look at the research data lifecycle. Does the cycle track how you have dealt with your data – or how you envision doing so? Do you think these steps actually form a cycle? Are there steps that you hadn’t fully considered? Do you think there are steps missing? If you now can see how your research data might have a “lifecycle,” will that change anything about your workflow going forward?
- show solution
- Answers to these questions may vary depending upon how scholars conceive of the research process and how they collect and analyze data. One aspect you might question is the arrow from “long-term management” to “discovery and planning”. Do you see one research project tightly linked to the next in the way this arrow suggests? One aspect you might not have thought about before is long-term management of your data; had you considered the possibility of (someone) taking care of your data for years or decades? A core concern with the RDL model, particularly in the context of qualitative research, is that it has gaps and glosses over details. You can probably think of multiple steps you will take between any two steps in the model. Also, there is not always a neat distinction between planning, initial, and final data collection. You (will) likely also shift between planning your research (including data collection), carrying it out, and writing. And qualitative researchers often go back and forth between data and theory. The lifecycle doesn’t reflect any of these “back-and-forths”. When you’re planning your research, you should.
Managing Research Data Through Their Lifecycle
This course follows your research data through their lifecycle, discussing important data management tasks that you should carry out in every phase of the RDL. The rest of Module 1 focuses on the crucial planning stage: you learn how to write an effective data management plan (DMP), and how to craft a consent script for human participant research that will allow you to share your data. Module 2 focuses on managing your data while you are collecting them. You will learn how to document your data so that they are easy to use in the future. You will also be presented with some practical strategies for organizing your data and files, including naming conventions and back-up strategies. Finally, you will learn how to transform the raw data you collect, e.g., how to digitize documents or transcribe interviews. Module 3 addresses sharing your data safely and responsibly. Module 4 considers writing and publishing with qualitative data.
Throughout this course, we will use a small image of the data lifecycle to locate in what phase you would carry out the tasks being discussed.